Research Article | Vol. 6, Issue 1 | Journal of Clinical Medical Research | Open Access |
Integrating Artificial Intelligence and Neural Networks for Enhanced Diagnosis of Atopic Dermatitis
Mahajabeen Madarkar1*
1Professor and Head of the Dermatology Department, SR Patil Medical College, Badagandi, Bagalkot, India
2Assistant Professor Physiology Department, SR Patil medical college, Badagandi, Bagalkot, India
3Medical Intern, Himalayan Institute of Medical Sciences, Jollygrant, Dehradun, Uttarakhand, India
*Correspondence author: Mahajabeen Madarkar, Professor and Head of the Dermatology Department, SR Patil Medical College, Badagandi, Bagalkot, India; Email: [email protected]
Citation: Madarkar M, et al. Integrating Artificial Intelligence and Neural Networks for Enhanced Diagnosis of Atopic Dermatitis. Jour Clin Med Res. 2025;6(1):1-9.
Copyright© 2025 by Madarkar M, et al. All rights reserved. This is an open access article distributed under the terms of the Creative Commons Attribution License, which permits unrestricted use, distribution, and reproduction in any medium, provided the original author and source are credited.
Received 05 April, 2025 | Accepted 21 April 2025 | Published 28 April, 2025 |
Abstract
Introduction: Atopic dermatitis, a chronic inflammatory skin condition, disproportionately affects young children. Statistics show that up to 90% of cases are diagnosed before the age of five. Despite its prevalence, the exact cause of this condition remains a mystery, frustrating both patients and healthcare professionals. This article delves into the exciting potential of Artificial Intelligence (AI) methods in diagnosing atopic dermatitis in this particularly vulnerable age group. We’ll explore how AI can be used to improve diagnostic accuracy and efficiency, potentially leading to earlier intervention and better management of the condition.
Methods: Atopic dermatitis, particularly prevalent among children under the age of five, remains a condition without a clearly defined etiology. This study investigates the application of artificial intelligence-specifically an Artificial Neural Network (ANN) for the diagnosis of atopic dermatitis in early childhood. The model incorporates input parameters including age, gender, presence of asthma, skin pH levels, dermoscopic findings and Immunoglobulin E (IgE) levels to enhance diagnostic accuracy.
Results: The research focuses on developing and evaluating an artificial neural network designed for the automated detection of Atopic Dermatitis (AD). Utilizing age, gender, asthma status, skin pH, dermoscopy and IgE levels, this approach has the potential to improve AD diagnosis accuracy, paving the way for similar models to further enhance future diagnostics.
Conclusion: This paper demonstrates a successful Artificial Neural Network for distinguishing atopic dermatitis from healthy skin, potentially impacting millions with this common condition.
Keywords: Atopic Dermatitis; Artificial Intelligence (AI); Neural Networks; Atopic Eczema; Atopy; Dermoscopy
Introduction
Atopic dermatitis, also known as eczema, is a chronic inflammatory skin condition that primarily affects children, especially those under the age of five [1-20]. This condition is characterized by dry, itchy skin and the appearance of red rashes, which can significantly disrupt a child’s daily life, including their ability to sleep, engage in physical activities and participate in social interactions [1,2,11]. The intense itching and skin irritation often lead to constant scratching, which can exacerbate the condition and cause secondary infections, further complicating management [5].
Although some children may eventually outgrow eczema as they age, the condition can persist into adulthood for others. This persistence can vary in severity, with some individuals experiencing occasional flare-ups while others may have continuous symptoms throughout their lives [2,20]. While the exact cause of atopic dermatitis remains elusive, it is widely believed to result from a combination of genetic predisposition and environmental triggers. Genetic factors are thought to affect the skin’s ability to retain moisture and protect against irritants and allergens, thereby making individuals more susceptible to developing the condition [3,17]. Several studies have identified specific genes related to immune response and skin barrier function that may contribute to the development of atopic dermatitis [21].
In addition to genetic factors, environmental triggers also play a crucial role in the onset and exacerbation of atopic dermatitis. For instance, individuals with a family history of eczema, asthma or other allergic conditions are at a significantly higher risk of developing the disease. This hereditary link is thought to involve a dysregulated immune response that causes the skin to react excessively to various environmental stimuli. Common environmental triggers for eczema flare-ups include dry weather, which can cause the skin to lose moisture and become more prone to irritation and irritants such as soaps, detergents and fragrances that can strip the skin of its natural oils. Allergens like dust mites, pet dander and pollen are also known to trigger flare-ups, especially in individuals who have heightened sensitivity to these substances [3].
Furthermore, environmental pollutants, food allergens and stress are additional factors that may contribute to the worsening of atopic dermatitis symptoms [16,19]. Managing the condition often involves a combination of strategies, including moisturizing the skin to maintain its barrier function, avoiding known irritants, and, in some cases, using topical corticosteroids or other treatments to control inflammation and itching [4,13].
As research continues to investigate the underlying causes of atopic dermatitis, new therapeutic approaches, such as biologic drugs that target specific immune pathways, are being developed [22]. Early diagnosis and intervention remain essential in managing the condition and improving the quality of life for individuals affected by atopic dermatitis.
Symptoms and Causes
Atopic dermatitis, also known as eczema, manifests with a range of distinctive symptoms [1,4]. Intense and persistent itching, often leading to irritation from scratching, is a hallmark feature [4]. The affected skin typically appears red, inflamed and may even ooze or crust over. Individuals with this condition experience dry, sensitive skin that may become scaly, cracked or flake. During flare-ups, small raised bumps or fluid-filled blisters may develop. Prolonged inflammation and scratching can lead to thickened skin, particularly in frequently scratched areas [5]. These symptoms, often beginning in childhood and persisting into adulthood, significantly impact the quality of life for those affected by this chronic and inflammatory skin condition [2].
Atopic dermatitis has a strong hereditary component. Individuals with a family history of atopic conditions like eczema, asthma or hay fever are significantly more likely to develop it. Specific genes associated with skin barrier function and immune response play a crucial role [1,6]. For example, mutations in the filaggrin gene have been extensively studied and are associated with an increased risk [6,12]. While genetics and immune system dysfunction play a major role in atopic dermatitis, environmental factors can also act as triggers. Common triggers include dry weather, exposure to dust mites and allergens, harsh soaps and detergents and certain fabrics like wool [3]. These triggers can exacerbate existing symptoms and contribute to flare-ups.
Artificial Intelligence
Artificial intelligence, as a branch of computer science, deals with the analysis of complex medical data. Neural networks have shown potential in aiding diagnosis, guiding treatment decisions and forecasting outcomes across various clinical situations [23]. In dermatology specifically, their application offers benefits like quicker data acquisition and faster result generation, which contribute to the streamlined development of effective treatment strategies [7].
Clinical features of atopic dermatitis are lesions distributed on the skin that can be of different morphology, intensity and duration, which is an aggravating circumstance for diagnosis [8].
Multiphoton Tomography (MPT) is frequently employed in these contexts, as it offers a non-invasive and label-free method for performing optical biopsies [14,15]. This paper aims to highlight how artificial intelligence can be applied in diagnosing atopic dermatitis, potentially enabling quicker diagnostic processes and more prompt treatment initiation [9,10].
Ethical Statement
The project did not meet the definition of human subject research under the purview of the IRB according to federal regulations and therefore, was exempt.
Methodology
Dataset Benchmarks:
For the development of the Artificial Neural Network (ANN), a dataset consisting of 500 samples was used, each categorized into one of two groups:
- Healthy subjects
- Subjects with atopic dermatitis
The dataset includes six key input parameters based on medical indicators of atopic dermatitis:
- Age: The age of the individual (in years)
- Gender: The biological sex of the individual (Male/Female)
- Presence of Asthma: Whether the individual has a history of asthma (Yes/No)
- Skin pH: The pH level of the skin, measured using a skin pH meter
- Dermoscopy: Clinical skin examination using dermoscopy to observe lesions
- IgE: Immunoglobulin E (IgE) levels, a marker for allergic responses
The output of the model is categorical, represented as either 0 (healthy subjects) or 1 (subjects with atopic dermatitis).
The output of the model is categorical, represented as either 0 (healthy subjects) or 1 (subjects with atopic dermatitis).
Age (Years) | Gender (Male/Female) | Percentage (%) |
1-5 | 98 Male 123 Female | 41.88% 46.24% |
5-15 | 95 Male 103 Female | 40.59% 38.72% |
15-25 | 33 Male 29 Female | 14.10% 10.93% |
25-30 | 8 Male 11 Female | 3.41% 4.13% |
TOTAL | 234 Male 266 Female | 100% 100% |
Table 1: Parameters based on medical indicators.
Class 0 | Class 1 | Total | |
Training | 90 | 320 | 410 |
Testing | 10 | 80 | 90 |
Total | 100 | 400 | 500 |
Table 2: The output of the model is categorical atopic dermatitis.
Artificial Neural Network Development (Fig. 1)
Figure 1: All parameters representation.
Result
For the purposes of this study, an Artificial Neural Network was designed to enable the automated diagnosis of atopic dermatitis.
The Evolving Role of AI in Skin Disease Diagnosis and Management
This study exemplifies the growing focus on deep learning algorithms and neural networks in diagnosing atopic dermatitis [7,8]. These models, alongside future advancements, hold promise for improving the accuracy of AD severity scoring. This translates to earlier and more precise treatment responses for patients, potentially leading to better treatment compliance through improved patient communication (Fig. 2-4) [9].
Broader Applications of AI in Skin Care
Given that the skin is our largest organ, Artificial Neural Networks (ANNs), Convolutional Neural Networks (CNNs) and other deep learning algorithms can play a significant role in diagnosing various skin conditions [18]. Their application can assist doctors in selecting the most effective treatment strategies, potentially reducing the reliance on a trial-and-error approach often encountered in skin disease management [10].
Figure 2: Dennie-morgan infraorbital fold in patient of atopic dermatitis.
Figure 3: Chelitis in patient of atopic dermatitis.
Figure 4: Anterior neck folds in patient of atopic dermatitis.
Discussion
Atopic dermatitis is a complex, chronic inflammatory skin condition that stems from both genetic and immunological factors. One of the core contributors to disease pathology is the dysfunction of the skin barrier, often linked to mutations in the filaggrin gene [12]. Filaggrin is critical in maintaining skin hydration and structural integrity and its deficiency results in a weakened barrier, making the skin more susceptible to environmental allergens and irritants [12,17]. This compromised barrier not only leads to increased dryness and sensitivity but also facilitates the onset and progression of inflammation [21].
This immune imbalance results in heightened levels of inflammatory cytokines, which exacerbate the itching, redness and irritation that define atopic dermatitis [4].
Furthermore, diagnostic challenges remain a significant issue, particularly for early-career clinicians. Differentiating between dermatological conditions such as atopic dermatitis, psoriasis and eczema can be difficult due to their overlapping visual features. To bridge this diagnostic gap, this study proposes a deep learning model that can analyse clinical skin images and accurately classify skin diseases [23]. By automating the diagnostic process, this tool offers valuable support to healthcare providers, reducing the likelihood of misdiagnosis and enabling timely, targeted treatment [9,10].
In summary, the combination of genetic susceptibility, immune dysregulation and environmental triggers contributes to the multifaceted nature of atopic dermatitis [3]. The integration of artificial intelligence into dermatological diagnostics holds promising potential to improve early detection, reduce diagnostic errors and ultimately enhance patient outcomes [24].
experienced and early-career dermatologists are especially prone to diagnostic mistakes, as conditions like psoriasis, eczema and atopic dermatitis are often easily confused. To address this issue and provide support to clinicians, this study introduces an end-to-end deep learning model that utilizes clinical skin images to automatically diagnose these dermatological conditions [23].
Conclusion
Atopic dermatitis or eczema, is a common skin condition that can be difficult to diagnose accurately due to symptom overlap with other dermatological disorders. This often leads to delays in treatment and prolonged patient discomfort. This study introduces an Artificial Neural Network (ANN) model capable of distinguishing atopic dermatitis from healthy skin with promising accuracy. By automating key aspects of diagnosis, the model has the potential to streamline clinical decision-making and improve early detection.
However, its current application is limited to binary classification—healthy skin versus atopic dermatitis. To increase its clinical value, the model should be expanded to include data from a variety of other skin conditions. Doing so would enable more precise differential diagnosis, helping clinicians identify and manage skin disorders more efficiently and accurately.
In conclusion, expanding the ANN’s scope could significantly enhance its diagnostic utility, offering faster, more reliable support for healthcare providers and better outcomes for patients.
Conflict of Interest
The authors declared no potential conflicts of interest with respect to the research, authorship and/or publication of this article.
Acknowledgement
Acknowledge those who provided support during the study.
Consent to Participate
The authors certify that they have obtained all appropriate patient consent.
Financial Disclosure
This research did not receive any grant from funding agencies in the public, commercial or not-for-profit sectors.
Data Availability
Data is available for the journal. Informed consents were not necessary for this paper.
Author’s Contribution
The authors contributed equally.
References
- Kolb L, Ferrer-Bruker SJ. Atopic dermatitis. StatPearls Publishing. 2025.
- Silverberg JI. Persistence of childhood eczema into adulthood. JAMA Dermatol. 2014;150(6):591-2.
- Jackson DS. Environmental triggers of atopic dermatitis: A systematic review of the literature. J Am Acad Dermatol. 2016; 75(2):446-53.
- Palmer S. Atopic dermatitis: Disease features, therapeutic options and a primer for clinicians. J Clin Med. 2021;10(6):1342.
- Rinaldi G. The itch-scratch cycle: A review of the mechanisms. Dermatol Pract Concept. 2019;9(2):90-7.
- Weidinger S, Beck LA, Bieber T, Kabashima K, Irvine AD. Atopic dermatitis in Kanerva’s occupational dermatology. Springer Berlin Heidelberg. 2012;1:201-12.
- Adam A, Ibrahim Z, Shapiai MI, Chew LC, Jau LW, Khalid M, et al. A two-step supervised learning artificial neural network for imbalanced dataset problems. Int J Innovative Computing, Information and Control. 2012;8(5):3163-72.
- Adam A, Shapiai I, Ibrahim Z, Khalid M, Chew LC, Jau LW, et al. A modified artificial neural network learning algorithm for imbalanced data set problem. In2010 2nd International Conference on Computational Intelligence, Communication Systems and Networks 2010:44-8.
- Bang CH, Yoon JW, Ryu JY, Chun JH, Han JH, Lee YB, et al. Automated severity scoring of atopic dermatitis patients by a deep neural network. Scientific Reports. 2021;11(1):6049.
- Bobrova M, Taranik M, Kopanitsa G. Using neural networks for diagnosing in dermatology. Health IOS Press. 2019;211-6.
- Camfferman D, Kennedy JD, Gold M, Martin AJ, Winwood P, Lushington K. Eczema, sleep and behavior in children. J Clin Sleep Medicine. 2010;6(6):581-8.
- Čepelak I, Dodig S, Pavić I. Filaggrin and atopic march. Biochemia Medica. 2019;29(2):214-27.
- Eichenfield LF, Tom WL, Chamlin SL, Feldman SR, Hanifin JM, Simpson EL, et al. Guidelines of care for the management of atopic dermatitis: section 1. Diagnosis and assessment of atopic dermatitis. J Am Acad Dermatol. 2014;70(2):338-51.
- Guimarães P, Batista A, Zieger M, Kaatz M, Koenig K. Artificial intelligence in multiphoton tomography: Atopic dermatitis diagnosis. Scientific Reports. 2020;10(1):7968.
- König K. Clinical in vivo multiphoton FLIM tomography. Methods and Applications in Fluorescence. 2020;8(3):034002.
- Nutten S. Atopic dermatitis: Global epidemiology and risk factors. Annals of Nutrition and Metabolism. 2015;66(1): 8-16.
- Ousey K, Cutting KF, Rogers AA, Rippon MG. The importance of hydration in wound healing: Reinvigorating the clinical perspective. J Wound Care. 2016;25(3):122-30.
- Holmes J, Sacchi L, Bellazzi R. Artificial intelligence in medicine. Ann R Coll Surg Engl. 2004;86:334-8.
- Sicherer SH, Leung DYM. Advances in allergic skin disease, anaphylaxis and hypersensitivity reactions to foods, drugs and insects in 2009. J Allergy and Clinical Immunol. 2010;125(1):85-97.
- Silverberg NB. A practical overview of pediatric atopic dermatitis, part 1: Epidemiology and pathogenesis. Cutis. 2016;97(4):267-71.
- Silverberg NB, Silverberg JI. Inside out or outside in: Does atopic dermatitis disrupt barrier function or does disruption of barrier function trigger atopic dermatitis. Cutis. 2015;96(6):359-61.
- Wood E, Shields BE. Use of complementary alternative medicine and supplementation for skin disease. Cutis. 2021;108(2):78-83.
- Wu H, Yin H, Chen H, Sun M, Liu X, Yu Y, et al. A deep learning, image based approach for automated diagnosis for inflammatory skin diseases. Annals of Translational Medicine. 2020;8(9):581.
- Liu Y, Jain A, Eng C, Way DH, Lee K, Bui P, et al. A deep learning system for differential diagnosis of skin diseases. Nature Medicine. 2020;26(6):900-8.
Author Info
Mahajabeen Madarkar1*
1Professor and Head of the Dermatology Department, SR Patil Medical College, Badagandi, Bagalkot, India
2Assistant Professor Physiology Department, SR Patil medical college, Badagandi, Bagalkot, India
3Medical Intern, Himalayan Institute of Medical Sciences, Jollygrant, Dehradun, Uttarakhand, India
*Correspondence author: Mahajabeen Madarkar, Professor and Head of the Dermatology Department, SR Patil Medical College, Badagandi, Bagalkot, India; Email: [email protected]
Copyright
Mahajabeen Madarkar1*
1Professor and Head of the Dermatology Department, SR Patil Medical College, Badagandi, Bagalkot, India
2Assistant Professor Physiology Department, SR Patil medical college, Badagandi, Bagalkot, India
3Medical Intern, Himalayan Institute of Medical Sciences, Jollygrant, Dehradun, Uttarakhand, India
*Correspondence author: Mahajabeen Madarkar, Professor and Head of the Dermatology Department, SR Patil Medical College, Badagandi, Bagalkot, India; Email: [email protected]
Copyright© 2025 by Madarkar M, et al. All rights reserved. This is an open access article distributed under the terms of the Creative Commons Attribution License, which permits unrestricted use, distribution, and reproduction in any medium, provided the original author and source are credited.
Citation
Citation: Madarkar M, et al. Integrating Artificial Intelligence and Neural Networks for Enhanced Diagnosis of Atopic Dermatitis. Jour Clin Med Res. 2025;6(1):1-9.
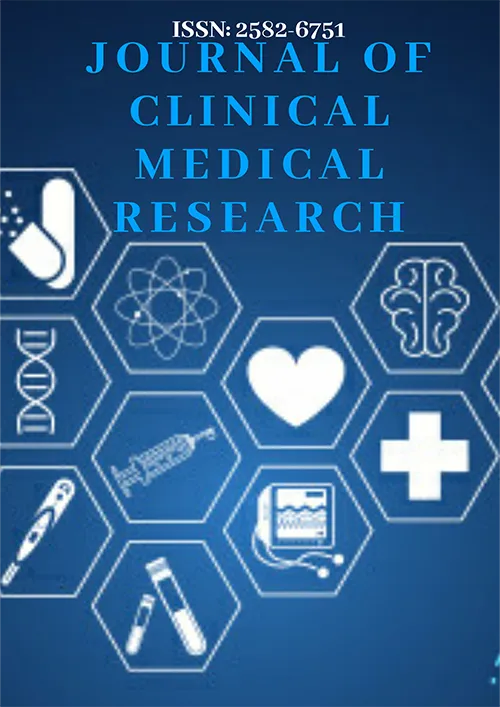