Home » Predictors of COVID-19 Related Fatalities during the First Three Months of the Pandemic: An Ecological Study of 114 Countries
Research Article | Vol. 4, Issue 1 | Journal of Clinical Medical Research | Open Access |
Predictors of COVID-19 Related Fatalities during the First Three Months of the Pandemic: An Ecological Study of 114 Countries
Andreas Katsimpris1,2*, Nafsika Voulgari1, Nikolaos Georgiadis3, Aristotelis Karamaounas1, Anna Maria Sideri1, John Katsimpris3, Stylianos Kandarakis1
1First Ophthalmology Department, “G.Gennimatas” Hospital, National and Kapodistrian University of Athens, Athens, Greece
2Department of Family and Community Medicine, School of Medicine, National and Kapodistrian University of Athens, “Ippokrateio” General Hospital of Athens, Athens, Greece
3Department of Ophthalmology, General Hospital of Patras “Agios Andreas”, Patras, Greece
*Corresponding Author: Andreas Katsimpris, First Ophthalmology Department, “G.Gennimatas” Hospital, National and Kapodistrian University of Athens, Leof. Mesogeion 154, Athens, Greece; Email: [email protected]
Citation: Katsimpris A, et al. Predictors of COVID-19 Related Fatalities during the First Three Months of the Pandemic: An Ecological Study of 114 Countries. Jour Clin Med Res.. 2023;4(1):1-7.
Copyright© 2023 by Katsimpris A, et al. All rights reserved. This is an open access article distributed under the terms of the Creative Commons Attribution License, which permits unrestricted use, distribution, and reproduction in any medium, provided the original author and source are credited.
Received 18 Dec, 2022 | Accepted 06 Jan, 2023 | Published 13 Jan, 2023 |
Abstract
Background: Coronavirus disease 2019 (COVID-19) has costed the lives of more than 2 million people during the first wave of the pandemic with the number of fatalities varying among different countries. Thus, we aimed to assess the associations of specific demographic, clinical and political variables with COVID-19 related fatalities in various countries, during the first three months of the pandemic.
Methods: We analyzed publicly available data from 192 regions (114 countries) and built a log-linear regression model with random intercepts in order to identify predictors of COVID-19 fatalities during the first three months of the pandemic. We used country-level data including total population, the percentage of people aged 65 and above, number of ICU beds per 100,000 people, geographical latitude and number of days from the first confirmed COVD-19 case to establishment of specific preventive control measures as explanatory variables of our statistical model.
Results: In our multivariate statistical model, one unit increases in the total population (in 10,000,000 units), percentage of population aged 65 and above and the number of days from the first confirmed COVID-19 case to the imposition of preventive measures, were related to 3.8% (95% CI: 0.8% to 6.9%), 7.1% (95% CI: 0.9% to 13.6%) and 1.8% (95% CI: 0.3% to 3.6%), respectively, higher number of COVID-19 related deaths.
Conclusion: Our findings imply positives associations of total population, percentage of population aged 65 and above and number of days from the first confirmed COVID-19 case to the imposition of preventive measures with COVID-19 fatal cases, during the first three months of the pandemic. Future non-ecological studies are warranted to confirm our results.
Keywords: COVID-19; Ecological Study; Prediction Model
Introduction
On 31 December 2019, the World Health Organization (WHO) was informed about a cluster of 27 patients with pneumonia of unknown etiology, who were identified in Wuhan city, Hubei province of China [1]. The causative agent was identified as a novel beta-coronavirus on 7 January 2020 by the Chinese authorities and WHO named the disease Coronavirus Disease 2019 (COVID-19) [2,3]. During its first wave, COVID-19, has infected over 100 million people around the globe with more than 2,000,000 deaths with WHO characterizing it as a pandemic on 12 March 2019 [4].
During the first wave of the pandemic, most of countries all over the world put into effect specific preventive measures in order to slow and stop the transmission of COVID-19. The preparedness of each country regarding the management of the COVID-19 varied, with some countries having a quicker and more effective response than others [5]. Moreover, with COVID-19 having been a global public health emergency, over the last 2 years research has focused on various aspects of this novel disease, trying to provide essential clinical and epidemiological information [6]. The main goal of this information has been to assist clinicians and public health authorities in the management of this disease. Various organizations, like the John Hopkins Center for Systems Science and Engineering (CSSE), have also provided open access to country-level data on COVID-19 cases which are updated on a daily basis [7,8]. The accessibility of this data has given the opportunity to researchers to identify any potential country-level sociodemographic, health care-associated and environmental parameters that could explain the variation of confirmed COVID-19 related deaths among countries [9].
Therefore, we conducted an ecological study with publicly available data on COVID-19 cases during the first three months of the COVID-19 pandemic. The major objective of this study was to access the associations of the percentage of people aged 65 and above, number of Intensive Care Unit (ICU) beds per 100,000 people, total population, geographical latitude, and number of days from the first confirmed COVD-19 case to establishment of specific preventive control measures, with the number of COVID-19 related deaths in various countries, in order to find any significant predictors of COVID-19 related deaths during the first three months of the pandemic.
Materials and Methods
Data Collection
This was a closed loop, two cycle audit and the population of the study was all the patients in our primary care department in This was an ecological study of country-level predictors of COVID-19 related deaths. In order to acquire the necessary data for our analysis we utilized various publicly available data sources. In our statistical model the outcome was number of COVID-19 related deaths in the selected countries after 3 months from the first confirmed COVID-19 case and the corresponding data was acquired from the COVID-19 data repository of the CSSE, Johns Hopkins University [7]. The explanatory variables of our statistical model for each country were: total population, the percentage of people aged 65 and above, number of ICU beds per 100,000 people, geographical latitude and number of days from the first confirmed COVD-19 case to establishment of specific preventive control measures.
More specifically, the total population and the percentage of people aged 65 and above of each country were acquired from the World Bank data repository which contains data from the 2019 Revision of World Population Prospects, United Nations Population Division the International Database, U.S. Census Bureau the Statistics and Demography Programme, Secretariat of the Pacific Community the Demographic Statistics, Eurostat and the census reports and other statistical publications from national statistical offices [10-14].
Data regarding the number of ICU beds per 100,000 people were obtained from already published articles [15-17]. For countries where we could not obtain any data on the number of ICU beds per 100,000 people, we used an indirect method of calculating it. First, we obtained for each country the number of total hospital beds per 100,000 people from the Global Health Observatory data repository [18]. Second, we calculated the percentage of the number of ICU beds to the number of total hospital beds per 100,000 people, only for the countries, for which we obtained from the research literature a valid number of ICU beds. Then, we multiplied this percentage with the number of total hospital beds per 100,000 people for each country to calculate an indirect estimate of ICU beds.
The geographical latitude of each country was reported in angular degrees from 0° at the equator to ±90° (90° at the North Pole and -90° at the South Pole) and was obtained from the COVID-19 data repository of the CSSE, Johns Hopkins University [7]. The exact date of the first confirmed COVID-19 case of each country was derived from the same database.
Moreover, we complemented our dataset in respect to days from the first reported confirmed COVID-19 case to the initiation of COVID-19 preventive measures. The specific preventive measures that we took into account were the following: 1) inspection measures at the entry points of each country, before the report of any COVID-19 cases, for people coming from countries with confirmed COVID-19 cases e.g. temperature screening in passengers for inbound flights, with subsequent isolation of confirmed cases at shelters provided by the government, 2) widespread laboratory testing and rigorous quarantine measures, including the utilization of high-tech resources to confirm self-quarantine and for contact tracing track, at the first 2 weeks from the first confirmed COVID-19 case, 3) shutting down of all non-essential places like bars, nightclubs, restaurants, cinemas and coffee shops, 4) ban on public gatherings of 10 or more people, 5) imposition of curfew on a national or sub-national level. We did not include the individual closure of educational institutes in our specific preventive safety measures since the number of times people come into close contact with each other does not significantly change for the majority of population, despite this measure. Data on the exact dates of implementation in each country of the preventive measures mentioned above were acquired through reports of governmental departments and news resources.
Finally, for 4 countries, China, U.S., Australia and Germany, data were available on the level of their administrative divisions (federal states or provinces) and thus these divisions were included with the remaining countries in the analysis. We acquired the necessary data for each state/province from census reports and statistical publications of national statistical offices [19-21].
Eligibility criteria for a country to be included in our ecological study required the country to have reported: 1) at least 100 confirmed COVID-19 cases, 2) at least 5 COVID-19 related deaths and 3) to have passed at least 90 days from the first COVID-19 case until the analysis of the data. The reasons for the selection of these specific inclusion criteria were to exclude any country that the spread of COVID-19 was in early stages and also to limit the possibility of including countries with severe underreport of COVID-19 data. Any country/region with missing data on either the outcome or the explanatory variables were excluded from the analysis.
Statistical Analysis
We used Pearson correlation coefficients to express the crude correlation among the continuous explanatory variables of our statistical model. Moreover, built a random-effects log-linear regression model in order to assess the associations between percentage of people aged 65 and above, number of ICU beds per 100,000 people, total population, geographical latitude, number of days since the first confirmed COVID-19 case and number of days from the first confirmed COVD-19 case to imposition of specific preventive control measures, with the number of deaths in various countries. An exponential behavior for the accumulated reported COVID-19 related deaths is assumed at the onset of the epidemy, and that’s the main reason we selected log-linear instead of simple linear regression model. Additionally, the number of COVID-19 related deaths in our dataset was log-normally distributed. The random effects method was selected to account for the observed variability of our outcome among countries and the random effects were expressed as random intercepts for each country.
We specified the following log-linear regression model with random intercepts:
log(μi) = β0 + β1*(percentage of people aged 65 and above) + β2*(total population) + β3*(number of ICU beds per 100,000 people) + β4*(geographical latitude) + β5*(number of days from the first confirmed COVD-19 case to imposition of specific preventive control measures) + ui, where μ ist he geometric mean of our outcome, β are the regression coefficients of our explanatory variables and i (i=1,…..,114) is the index of each country. We built a fully adjusted model that includes all the explanatory variables and 5 unadjusted models for each of our explanatory variables and the associations with our outcome were reported as ratios of the number of COVID-19 related deaths per one-unit increase in the explanatory variables, by exponentiations the calculated regression coefficients.
The regression estimates are reported with their corresponding 95% confidence intervals (95%CI) and p-values derived from two-sided statistical tests of significance. We set the significance probability level α at 0.05, and a p-value less than that was considered statistically significant. The assumptions of linear regression were tested. Data were analyzed using the statistical software R (version 3.5.1, Foundation for Statistical Computing, Vienna, Austria) and the analysis was completed on 4th March 2022.
Results
In our ecological study we included 192 regions (114 countries) with 337,760 COVID-19 related deaths in total (Supplementary Table 1). The mean (minimum-maximum value) of the total population, the percentage of people aged 65 and above, the number of ICU beds per 100,000 people, geographical latitude and the number of days from the first confirmed COVD-19 case to establishment of specific preventive control measures were 42,181,888 (33,785-1,352,617,328), 14.6 (1.1-27.6), 15.5 (1.0-63.0), 30.7 (-38.4-64) and 25.7 (0-90) respectively. There was no high correlation (Pearson coefficients > 0.8) among our continuous explanatory variables, ranging from -0.18 to 0.58 (Fig. 1).
In the univariate models ICU beds per 100,000 people and geographical latitude were not associated with the number of COVID-19 related deaths (Table 1). One unit increases in the total population (in 10,000,000 units), percentage of population aged 65 and above and the number of days from the first confirmed COVID-19 case to the imposition of preventive measures, were related to 4.2% (95% CI: 1.1% to 7.4%), 7.2% (95% CI: 1.4% to 13.4%) and 2.4% (95% CI: 1.0% to 4.0%), respectively, higher number of COVID-19 related deaths (Table 1).
In the multivariable model ICU beds per 100,000 people and geographical latitude still were not associated with the number of COVID-19 related deaths, while one unit increases in the total population (in 10,000,000 units), percentage of population aged 65 and above and the number of days from the first confirmed COVID-19 case to the imposition of preventive measures, were related to 3.8% (95% CI: 0.8% to 6.9%), 7.1% (95% CI: 0.9% to 13.6%) and 1.8% (95% CI: 0.3% to 3.6%) , respectively, higher number of COVID-19 related deaths (Table 1).
Figure 1. Correlation plot of explanatory variables of our analysis.
Variables | Unadjusted Model | 95% CI | P-value | Fully Adjusted Model | 95% CI | P-value |
Total population (in 10,000,000 units) | 1.042 | 1.011-1.074 | 0.008* | 1.038 | 1.008-1.069 | 0.002* |
Percentage of population aged ≥65 | 1.072 | 1.014-1.134 | 0.014* | 1.071 | 1.009-1.136 | 0.076* |
ICU beds per 100,000 people | 1.002 | 0.968-1.037 | 0.885 | 0.999 | 0.964-1.035 | 0.726 |
Geographical Latitude | 1.008 | 0.993-1.023 | 0.272 | 1.006 | 0.990-1.050 | 0.242 |
Days until imposition of preventive measures | 1.024 | 1.010-1.040 | 0.014* | 1.018 | 1.003-1.036 | 0.020* |
*Statistically significant associations (P-value<0.05) |
Table 1: Association estimates of COVID-19 related deaths and explanatory variables of our statistical model with corresponding 95% confidence intervals (95% CI).
Discussion
In this ecological study of 114 countries, we presented epidemiological evidence indicating that specific country and state-level variables can partially explain the variation of COVID-19 related fatalities. More specifically, our findings imply positives associations of total population, percentage of population aged 65 and above and number of days from the first confirmed COVID-19 case to the imposition of preventive measures with COVID-19 fatal cases.
The variation in COVID-19 related fatalities among countries has been documented by several studies during the pandemic [22,23]. Moreover, available data suggest that COVID-19 fatality rates are associated with a variety of factors including demographic, economic, political and clinical variables [22]. Most of these factors, like percentage of elderly population, share of population with comorbidities, GDP per capita, population size, number of hospital beds and percentage of GPD allocated to health care expenditure can explain to a significant degree the differences of COVID-19 fatality rates seen among countries. Furthermore, it has been observed that countries which promptly implemented preventive measures for diminishing the transmission of COVID-19 had fewer fatalities than those that delayed [22].
There are several possible explanations for our results. Although, in our study the number of ICU beds per 100,000 people was not a significant predictor of COVID-19 related deaths during the first months of the pandemic, several studies have shown that increased availability of ICU beds and hospital resources in general, could have an inverse association with COVID-19 mortality [24]. In addition to observational studies, simulation studies have also suggested the prevention of deaths due to COVID-19 by increasing the hospital critical care capacity limits [25]. These differences compared to our results may have occurred due to the fact we analyzed data only from the first months of the pandemic, where most of the health systems worldwide have been overwhelmed by the pandemic regardless of how sufficient their resources were. For the same reasons, our geographic latitude estimate may have been attenuated toward the null, although it has been shown that higher geographic latitude is associated with increased fatality due to COVID-19.
The generalizability of these results is subject to certain limitations. First of all, we assumed that reporting of COVID-19 confirmed and fatal cases were comparable among the 114 countries, something that may not be true. Second, we used an indirect method of estimating the ICU beds per 100,000 people in countries where we could not obtain any data on the number of ICU beds, which may have biased our associations. Third, there was great variability in the extend of how strict the preventive measures in each country were, making it difficult to decide the exact date of establishment of these measures and their effect of COVID-19 related deaths. Final, we should also highlight the possibility of ecological fallacy in our study, since we made inferences about individuals based on aggregate (country-level) data.
Conclusion
In conclusion, we found that total population, percentage of population aged 65 and above and the number of days from the first confirmed COVID-19 case to the imposition of preventive measures were associated with COVID-19 related deaths, using a multivariate regression model with data from 114 countries. Since the emergence of COVID-19 pandemic has caused a unique disruption in our daily lives, challenging not only the scientific community but also society in general, it would be necessary for further longitudinal studies with data based on individuals, larger sample sizes and objective measures of explanatory variables to assess these associations.
Conflict of Interest
The authors have no conflict of interest to declare.
Author Contributions
Andreas Katsimpris, Nafsika Voulgari and Nikolaos Georgiadis contributed to the conceptualization, methodology, data analysis, writing, review and editing of the manuscript. Stylianos Kandarakis and John Katsimpris contributed to the writing and editing of the manuscript. Andreas Katsimpris and Nafsika Voulgari contributed equally to the work and should be considered co-first authors.
References
- Lu H, Stratton CW, Tang YW. Outbreak of pneumonia of unknown etiology in Wuhan, China: The mystery and the miracle. J Med Virol. 2020;92(4):401-2.
- Huang C, Wang Y, Li X, Ren L, Zhao J, Hu Y, et al. Clinical features of patients infected with 2019 novel coronavirus in Wuhan, China. The Lancet. 2020;395(10223):497-506.
- WHO. Novel Coronavirus-China. 2020. [Last accessed on: January 06, 2023] https://www.who.int/csr/don/12-january-2020-novel-coronavirus-china/en/
- World Health Organization, Coronavirus disease 2019 (COVID-19) Situation Report – 72. [Last accessed on: January 06, 2023] https://www.who.int/docs/default-source/coronaviruse/situation-reports/20200401-sitrep-72-covid-19.pdf?sfvrsn=3dd8971b_2.
- Caristia S, Ferranti M, Skrami E, Raffetti E, Pierannunzio D, Palladino R, et al. Effect of national and local lockdowns on the control of COVID-19 pandemic: a rapid review. Epidemiol Prev. 2020;44(5-6 Suppl 2):60-8.
- Sharma A, Ahmad Farouk I, Lal SK. COVID-19: A Review on the Novel Coronavirus Disease Evolution, Transmission, Detection, Control and Prevention. Viruses. 2021;13(2).
- Novel Coronavirus (COVID-19) Cases, provided by JHU CSSE. [Last accessed on: January 06, 2023].https://github.com/CSSEGISandData/COVID-19
- EU Open Data Portal. [Last accessed on: January 06, 2023]. https://data.europa.eu/euodp/en/data/dataset/covid-19-coronavirus-data/resource/55e8f966-d5c8-438e-85bc-c7a5a26f4863
- Ting DSW, Carin L, Dzau V, Wong TY. Digital technology and COVID-19. Nat Med. 2020;26(4):459-61.
- The World Bank. [Last accessed on: January 06, 2023] https://data.worldbank.org/indicator/sp.pop.totl
- United Nations Population Division. World Population Prospects: 2019. [Last accessed on: January 06, 2023]. https://population.un.org/wpp/
- U.S. Census Bureau: International Database. [Last accessed on: January 06, 2023] https://www.census.gov/programs-surveys/international-programs/about/idb.html
- Secretariat of the Pacific Community: Statistics and Demography Programme. [Last accessed on: January 06, 2023] https://prism.spc.int/
- Eurostat: Demographic Statistics. [Last accessed on: January 06, 2023] https://ec.europa.eu/eurostat/web/population-demography-migration-projections/data
- Phua J, Faruq MO, Kulkarni AP, Redjeki IS, Detleuxay K, Mendsaikhan N, et al. Critical care bed capacity in Asian countries and regions. Crit Care Med. 2020.
- Rhodes A, Ferdinande P, Flaatten H, Guidet B, Metnitz PG, Moreno RP. The variability of critical care bed numbers in Europe. Intensive Care Med. 2012;38(10):1647-53.
- Wallace DJ, Angus DC, Seymour CW, Barnato AE, Kahn JM. Critical care bed growth in the United States. A comparison of regional and national trends. Am J Respir Crit Care Med. 2015;191(4):410-6.
- World Health Organization (WHO): Global Health Observatory data repository. [Last accessed on: January 06, 2023]. https://apps.who.int/gho/data/view.main.HS07v
- Gesundheitsberichterstattung des Bundes. [Last accessed on: January 06, 2023] http://www.gbe-bund.de/oowa921-install/servlet/oowa/aw92/dboowasys921.xwdevkit/xwd_init?gbe.isgbetol/xs_start_neu/&p_aid=3&p_aid=22255692&nummer=841&p_sprache=D&p_indsp=-&p_aid=39894203
- Statistisches Bundesamt. DESTATIS. [Last accessed on: January 06, 2023]. https://www.destatis.de/DE/Home/inhalt.html;jsessionid21AA2D8216543D9437D3585E157552C9.internet8732
- National bureau of statistics of China. [Last accessed on: January 06, 2023] http://www.stats.gov.cn/english/
- Sorci G, Faivre B, Morand S. Explaining among-country variation in COVID-19 case fatality rate. Sci Rep. 2020;10(1):18909.
- Ioannidis JPA. Global perspective of COVID-19 epidemiology for a full-cycle pandemic. Eur J Clin Invest. 2020;50(12):e13423.
- Janke AT, Mei H, Rothenberg C, Becher RD, Lin Z, Venkatesh AK. Analysis of hospital resource availability and COVID-19 mortality across the United States. J Hosp Med. 2021;16(4):211-4.
- Zebrowski A, Rundle A, Pei S, Yaman T, Yang W, Carr BG, et al. A spatiotemporal tool to project hospital critical care capacity and mortality from COVID-19 in US Counties. Am J Public Health. 2021;111(6):1113-22.
This work is licensed under a Creative Commons Attribution 2.0 International License.
Author Info
Andreas Katsimpris1,2*, Nafsika Voulgari1, Nikolaos Georgiadis3, Aristotelis Karamaounas1, Anna Maria Sideri1, John Katsimpris3, Stylianos Kandarakis1
1First Ophthalmology Department, “G.Gennimatas” Hospital, National and Kapodistrian University of Athens, Athens, Greece
2Department of Family and Community Medicine, School of Medicine, National and Kapodistrian University of Athens, “Ippokrateio” General Hospital of Athens, Athens, Greece
3Department of Ophthalmology, General Hospital of Patras “Agios Andreas”, Patras, Greece
*Corresponding Author: Andreas Katsimpris, First Ophthalmology Department, “G.Gennimatas” Hospital, National and Kapodistrian University of Athens, Leof. Mesogeion 154, Athens, Greece; Email: [email protected]
Copyright
Copyright© 2023 by Katsimpris A, et al. All rights reserved. This is an open access article distributed under the terms of the Creative Commons Attribution License, which permits unrestricted use, distribution, and reproduction in any medium, provided the original author and source are credited.
Citation
Citation: Katsimpris A, et al. Predictors of COVID-19 Related Fatalities during the First Three Months of the Pandemic: An Ecological Study of 114 Countries. Jour Clin Med Res.. 2023;4(1):1-7.
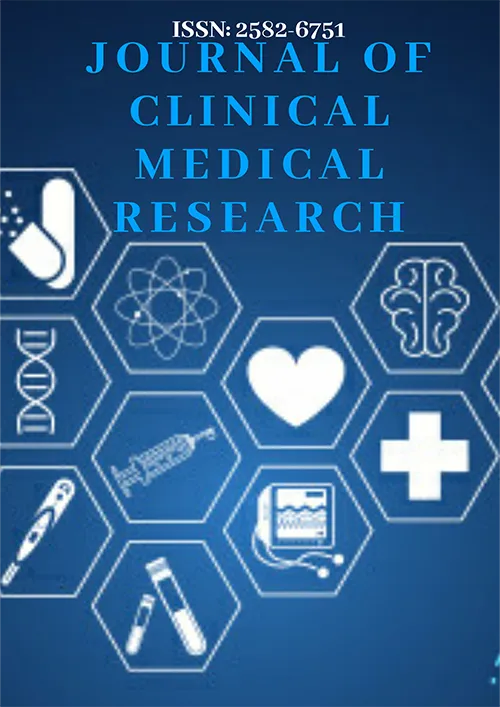