Home » Statistical Ethics in Medical Research: A Narrative Review
Review Article | Vol. 4, Issue 3 | Journal of Clinical Medical Research | Open Access |
Statistical Ethics in Medical Research: A Narrative Review
Thomas F Heston1*
1Department of Medical Education and Clinical Sciences, Washington State University, Spokane, USA and Department of Family Medicine, University of Washington, Seattle, USA
*Correspondence author: Thomas F Heston, Department of Medical Education and Clinical Sciences, Washington State University, Spokane, USA and Department of Family Medicine, University of Washington, Seattle, USA; Email: [email protected]
Citation: Heston TF. Statistical Ethics in Medical Research: A Narrative Review. Jour Clin Med Res. 2023;4(3):1-10.
Copyright© 2023 by Heston TF. All rights reserved. This is an open access article distributed under the terms of the Creative Commons Attribution License, which permits unrestricted use, distribution, and reproduction in any medium, provided the original author and source are credited.
Received 04 Nov, 2023 | Accepted 24 Nov, 2023 | Published 02 Dec, 2023 |
Abstract
Bioethics necessitates the meticulous planning, application and interpretation of statistics in medical research. However, the pervasive misapplication and misinterpretation of statistical methods pose significant challenges. Common errors encompass p-hacking, misconceptions regarding statistical significance, neglecting to address study limitations and failing to evaluate data fragility. Historically, such statistical missteps have led to regrettable and severe adverse health outcomes for society. For instance, prominent research on hormone replacement therapy likely resulted in an increased incidence of heart attacks, strokes and cardiovascular death in postmenopausal women, rectified only after the errors were identified. Likewise, past vaccine trials have oscillated between overemphasizing and underemphasizing side effects, resulting in public harm. This narrative review scrutinizes prevalent statistical errors and presents historical case examples. Recommendations for future research include: a) ethical review boards should incorporate a more rigorous evaluation of statistical methodologies in their assessment of clinical trial proposals; b) journals should mandate that research data become open-access rather than proprietary to allow for improved post-publication peer review; and c) in addition to addressing study limitations, articles should encompass a discussion of the ethical ramifications of their findings.
Keywords: Reproducibility; Research Ethics; Statistics; Medical Research; Reproducibility Crisis
Article Type
Review Article
Introduction
Biostatistics, applying statistical methods to biological, medical and public health research, is fundamental to rigorous scientific inquiry. By providing robust data analysis and interpretation frameworks, biostatistics ensures findings’ validity, reliability and generalizability and profoundly influences clinical and policy decisions [1].
The 18th century marked significant strides in probabilistic reasoning and controlled experiments. John Arbuthnot’s 1710 evaluation of birth statistics in London using probability was an early breakthrough when he found with high certainty that the birth rate for males was greater than that for females [2]. Concurrently, James Lind’s pioneering 1753 scurvy treatment experiment established essential foundations for controlled trials by incorporating randomization and accounting for confounders [3,4]. Later contributions came from mathematicians like Daniel Bernoulli, who applied statistical thinking to inoculation against smallpox and Pierre-Simon Laplace, renowned for developing early Bayesian inference in his 1814 seminal work, A Philosophical Essay on Probabilities [5,6]. These innovations demonstrated the growing power of statistics to produce actionable medical insights. Key 19th century figures include Florence Nightingale, who effectively applied statistics to demonstrate the critical role of sanitation and Francis Galton, renowned for developing statistical concepts like correlation and regression broadly applicable in biology [7,8].
The 20th century marked increased formalization of ethical guidelines for clinical trials via documents like the Nuremberg Code, the Declaration of Helsinki and the Belmont Report. Together, these guidelines underscored the need for rigorous statistical approaches to minimize harm and maximize benefits [9]. Karl Pearson and Ronald Fisher, two prominent statisticians of the 20th century, played pivotal roles in formalizing the calculation and interpretation of the p-value [10]. The advent of card tabulators and electronic computers marked a significant leap forward in statistical analysis capabilities [11]. These technological advancements laid the foundation for modern-day statistical methods that continue to shape the landscape of scientific research. The advent of electronic computers and sophisticated software in the late 20th century marked a pivotal leap forward, providing the computational power to develop and apply intricate statistical techniques like multivariate regression, advanced predictive modeling and real-time data analytics.
The evolution of biostatistics from Graunt’s 17th century contributions to the technological leaps of the 20th century demonstrates its indispensable role in ensuring scientific rigor and integrity, which are fundamental to the ethical conduct of medical research. This ever-advancing field provides a moral, analytical foundation for quality clinical trials.
As Pierre-Simon Laplace famously stated, “Probability theory is nothing but common sense reduced to calculation.” This succinctly captures how biostatistics brings rigor and structure to analyzing uncertainty. Florence Nightingale underscored the life-saving potential of biostatistics when she said, “To understand God’s thoughts, we must study statistics, for these are the measure of His purpose.” Finally, Ronald Fisher, a founder of modern statistical science, reminded researchers, “To call in the statistician after the experiment is done may be no more than asking him to perform a post-mortem examination: he may be able to say what the experiment died of”.
This narrative review article aims to discuss the pivotal role of statistics in upholding research integrity and to examine common statistical pitfalls that can undermine the reproducibility and ethical integrity of medical research.
Statistical Hazards in Biomedical Research
In current biomedical research, statistical analyses are pivotal in validating findings and drawing evidence-based conclusions. However, the ubiquity of high-powered computing platforms has facilitated the effortless calculation of intricate statistical algorithms, sometimes leading to inappropriate and overly complex applications of statistical tools. To avoid the most common statistical errors, researchers should continually review the fundamentals of basic statistics to understand potential pitfalls and how to address them [12,13]. The following sections discuss several pitfalls in biostatistics that may not only skew results but also raise ethical concerns due to improper statistical planning and analysis. This is not an exhaustive list or an in-depth summary of each hazard but rather an overview of multiple areas of biostatistics that can result in flawed research.
Over Reliance on Statistical Significance Versus Clinical Significance
Statistical significance is often misconstrued as indicative of clinical importance. Researchers frequently apply an arbitrary cut-off point of 5% (p < 0.05) to determine the “significance” of their findings [14]. Such an approach can be misleading and lead to suboptimal patient care when the focus should be on clinical significance instead. For example, a drug may show a statistically significant reduction in blood pressure but only by an average of 1 mmHg, which is clinically irrelevant.
Over-analysis of Data: Missing the Forest for the Trees
Another pitfall is the over-analysis of data, which can cause researchers to lose sight of the broader implications of their work. With advanced computational capabilities, subjecting data to numerous statistical tests and inappropriately selecting multiple variables becomes tempting [15]. However, this can result in “noise” overshadowing the “signal” thereby diluting the actual message or findings the research aims to convey.
Failure to Address Data Fragility
The reporting of statistical outcomes needs to mention how fragile or robust the data is. A study may tout significant findings, but the purported significance can be misleading if the results are based on fragile data sensitive to minor adjustments. Fragility indices should, therefore, be included to prevent over-hyping effects [16].
Neglecting Effect Size
A common issue arises when large sample sizes are employed: p-values often fall below 0.05, thus appearing statistically significant. The reason is that statistical analysis considers standard errors, calculated as the standard deviation divided by the square root of the sample size minus one. Standard errors shrink as the sample size grows and clinically irrelevant and statistically significant differences are more likely to be identified [17]. However, this can be associated with minuscule effect sizes, rendering the findings less impactful than the p-value might suggest [18].
P-Hacking: Manipulating Data to Achieve Significance
P-hacking or data dredging, is another concerning trend in biomedical research [19]. P-hacking involves manipulating data analysis to obtain statistically significant results, usually a p-value below 0.05. This can include techniques like testing many different combinations of variables, excluding specific data points and stopping analysis when significance is reached. These practices inflate Type I errors, the false positive rate and undermine the validity of the findings.
Ignoring Prevalence Rates
Even when the difference in population means is statistically significant, the overall prevalence rate in a given population can override this significance. For example, a statistically significant improvement in treatment outcomes may only apply to a tiny fraction of the patient population, making the finding less meaningful in broader clinical practice [20].
Over-Reliance on Models
Statistical models are valuable tools for simplifying complex biological phenomena. However, an unwarranted faith in models, especially without ongoing updates based on new data, can lead to substantial errors in interpreting and applying research findings [21]. The responsible and ethical use of models requires testing and validation before deployment.
The Multiple Comparisons Problem
When a data set is subjected to numerous statistical tests, the likelihood of identifying at least one “significant” result purely by chance increases. Without proper correction methods like the Bonferroni correction or the Benjamini-Hochberg procedure, the false discovery rate could be inflated, leading to incorrect conclusions [22].
Survivorship Bias
Survivorship bias occurs when researchers focus only on subjects that “survived” a process or passed a selection filter, neglecting those who did not. It is a reporting bias that can occur due to publication bias (only publishing statistically significant findings) or selective reporting of a visible subgroup that gets mistaken for representing the entire group [23]. This can skew results and conclusions, as the full range of data is not considered [24,25].
Not Accounting for Confounding Variables
Failure to account for confounding variables can lead to incorrect inferences about causal relationships. For example, if a study finds that drug A lowers blood pressure but fails to account for lifestyle changes like diet, the study’s conclusions might be inaccurate. This is a common cause for medical research that subsequently gets reversed. Randomization can help account for known and unknown confounding variables [26].
Autocorrelation
In analyzing time-series data, accounting for the likelihood of autocorrelation between measurements taken in close temporal proximity is imperative for researchers. Neglecting autocorrelation can result in invalid statistical tests since it violates the assumption of independence between variables. Overlooking this statistical characteristic can bias estimates and reduce precision, compromising the validity of subsequent scientific inferences. Specialized statistical techniques, including Seasonal AutoRegressive Integrated Moving Average (SARIMA) models and Nonlinear Autoregressive Neural Networks (NANN), are often employed to mitigate this. For example, SARIMA and NANN were utilized to predict new patient admissions to a hospital so resources could be better managed (27). They found that the linear model, SARIMA, combined with NANN, was best at predicting monthly trends, but NANN alone was better at predicting daily trends.
Heteroscedasticity
The assumption that the variance of the errors is constant across all levels of the independent variables is crucial for many statistical tests. Violations of this assumption (heteroscedasticity) can distort findings and weaken the reliability of hypothesis tests. The Harrison-McCabe test can be used to evaluate for heteroscedasticity [28].
Selection Bias
Selection bias occurs when the sample obtained does not represent the population intended for the study. For example, if a study on a drug’s effectiveness only includes healthy young adults, the results may not generalize to older populations or those with comorbid conditions. Sampling bias is one type of selection bias that can occur due to non-random sampling approaches that systematically exclude certain members of the target population. For instance, convenience sampling based on easily accessible subjects may bias the sample. Another cause can be the exclusive analysis of research subjects with complete datasets and throwing out those with missing data, which in the past was common with trauma research [29]. Because there is often a medical reason for missing data, this practice can skew the results, leading to incorrect conclusions from the research.
Collinearity
When two or more variables are highly correlated, it becomes difficult to separate the individual effects of these variables. This is particularly problematic in multivariate regression analyses [30].
Post-Hoc Rationalizations
After obtaining results, researchers might be tempted to explain unexpected findings with reasoning not part of the initial study design. While not always inappropriate, it can often be misleading and is generally considered poor scientific practice [31]. This practice can not only lead to poor medical care, but it can have legal ramifications. For example, using vague symptoms at a later date to predict child abuse at an earlier date can result in grave errors in legal decisions [32].
Simpson’s Paradox
Simpson’s Paradox occurs when a trend appearing in separate groups disappears or reverses when combined. It highlights the importance of stratified analysis to understand subgroup effects. Adjusting disease prevalence rates appropriately can help overcome this effect in many cases [20,33].
Peer-Review and Moral Hazards
Examining the role of pre-publication peer review in perpetuating certain statistical shortcomings is imperative. Although peer review is designed to enhance research quality and mitigate the spread of misinformation, the system has limitations and ethical concerns. These include potential biases and a disproportionate emphasis on statistically significant outcomes. The definition of a “peer” within this context exhibits considerable variability and reviewers frequently offer inconsistent feedback. The peer review process also manifests a notable “establishment bias” leading to differential treatment of research papers based on institutional affiliation [34].
The misapplication of statistical methods can directly violate ethical medical research principles. For example, p-hacking to achieve statistical significance when the actual effect size is negligible goes against the principle of beneficence. Though it may produce an impressive p-value, the clinical benefit to patients is likely minimal. Conversely, failing to account for confounding factors correctly can overestimate an intervention’s effectiveness, violating non-maleficence if it leads to patient harm. Not recognizing the fragility of findings could cause results to be over-generalized beyond what the data supports, undermining beneficence. While ethical research requires meticulous study design and execution, robust statistical practices provide the analytical framework to uphold these ethical obligations. Turning a blind eye to limitations, flexibility in data analysis and selective reporting may achieve publication, but at the cost of breaching principles meant to protect human subjects. An overview of common statistical issues that can result in significant errors are listed in Table 1.
Issue | Explanation |
Statistical Significance Versus Clinical Significance | Large sample sizes will frequently show statistically significant results that are clinically meaningless |
Over-analysis of Data: Missing the Forest for the Trees | Wide availability of statistical software can lead to over-analysis |
Failure to Address Data Fragility | Even when a result is statistically significant, it can be fragile |
Neglecting Effect Size | Numerical precision does not always correspond with clinical importance |
P-Hacking: Manipulating Data to Achieve Significance | If enough tests are done, the chances are that some will be statistically significant |
Ignoring Prevalence Rates | Large changes in a condition with low prevalence can distort actual significance |
Over Reliance on Models | Models simulate reality and are frequently wrong |
The Multiple Comparisons Problem | Multiple comparisons can lead to false positives |
Survivorship Bias | Only looking at survivors may miss important factors |
Confounding Variables | Biological systems are susceptible to multiple confounders |
Autocorrelation | The correlation of a variable with itself over time |
Heteroscedasticity | Variance of errors or the response variable is non-constant, leading to unreliable standard errors |
Selection Bias | The sample used may not be representative of the population, leading to biased results |
Collinearity | High correlation among independent variables can distort the estimated relationship |
Post-Hoc Rationalizations | Forming hypotheses after results are known can lead to misleading interpretations |
Simpson’s Paradox | A trend appears in several groups of data but disappears or reverses when these groups are combined |
Peer-Review and Moral Hazards | The peer review process can be influenced by biases or conflicts of interest, affecting the integrity of research |
Table 1: Statistical issues that can lead to data interpretation errors.
In summary, as the biomedical research community increasingly relies on statistical methodologies, vigilance is essential to avoid the misuse of statistical tools. Accurate, ethical research necessitates a nuanced understanding of the complex interplay between statistical and clinical significance, among other factors, to truly advance the field.
Case Studies
These case studies demonstrate the importance of statistics in ethical medical research. The misapplication of statistical planning and analysis can result in significant harm by coming to incorrect conclusions or a significant delay in medical advances.
Hormone Replacement Therapy
In a seminal study conducted in 1991, Hormone Replacement Therapy (HRT) was associated with reduced incidence rates of coronary heart disease among postmenopausal individuals undergoing estrogen therapy [35]. This observational investigation included a significant cohort of nearly 50,000 women from the Nurses’ Health Study (NHS). Over a decade-long follow-up, this research meticulously recorded 224 cases of stroke, 405 events of major coronary disease and 1,263 total fatalities. The study’s large sample size of nearly 50,000 participants provided considerable statistical power. Using multivariate regression to account for age, smoking status, cholesterol levels and other variables was a methodological strength in assessing the independent effect of HRT on heart disease risk. The relative risks were discerned by comparing participants who had undergone HRT and those who had not. A Cox proportional hazards model was rigorously employed to control for potential confounding variables. Within the NHS study, multivariate regression methods were utilized to account for various confounding variables, such as age (categorized in 5-year increments), cigarette smoking, hypertension, elevated serum cholesterol and a family history of myocardial infarction before age 60. While this methodological framework allowed for a detailed assessment of HRT’s impact on coronary heart disease, its limitations must be recognized. The exclusion of data on physical activity, even when available, might have introduced bias, given the known protective effects of exercise against heart disease. The dependence on self-reported information, especially regarding crucial variables like smoking habits and medical history, might have induced recall bias. The sample was fairly homogeneous, consisting primarily of caucasian nurses. Additionally, the categorization of age and the binary distinction of specific risk factors could have led to potential misclassification, impacting the research conclusions. However, it’s significant that HRT became the standard of care for postmenopausal individuals primarily based on this study’s results during the 1990s.
Subsequent research by the Women’s Health Initiative (WHI) in 2002 contradicted these earlier findings, determining that HRT was linked with an elevated cardiovascular risk [36]. This latter study employed a robust, randomized, placebo-controlled, double-blind methodology on a more diverse sample. As a result, HRT is no longer advocated as a preventive measure against cardiovascular disease.
The implications of the initial endorsement of HRT for postmenopausal individuals remain intricate. The impact was undoubtedly significant, given the visibility of the NHS article in the New England Journal of Medicine and its association with Harvard University. A more thorough examination of the statistical limitations inherent in the NHS study could have tempered the widespread, erroneous enthusiasm for HRT during the 1990s. Some of the issues with the original study included:
- In the 1991 NHS research, participants mainly consisted of registered nurses from 11 US states. Owing to their profession, these nurses likely had enhanced access to healthcare services and medical information compared to the broader female population of that era. This specific group might have introduced a selection bias, potentially affecting the study’s findings and applicability to a more diverse demographic
- The absence of randomization made it challenging to control for confounding factors effectively. Although data on numerous variables and outcomes were accumulated, the multivariate regression model omitted protective elements such as physical activity. Paired with the biennial reset of outcome analysis, this might have potentially led to statistical errors stemming from over-analysis and p-hacking [19]
- A salient takeaway from the evolution of HRT guidelines is the need for transparently addressing research limitations. Regrettably, the NHS research only provided a limited discussion on its research constraints, a pattern still prevalent in medical research. For instance, a dental literature review demonstrated that only 27% of randomized clinical trials incorporated discussions of study limitations [37]
From a statistical perspective, there are numerous ethical considerations to consider. First, observational studies suggesting significant shifts in medical therapy should be succeeded by more stringent randomized clinical trials. When adjusting an independent outcome, such as cardiovascular disease, by multivariate regression, it’s imperative to include risk and protective factors. The conclusions might not apply broadly if a study’s participants are relatively uniform. Finally, researchers should carefully examine and detail in their manuscripts the statistical limitations inherent in their investigations.
From a statistical perspective, there are numerous ethical considerations to consider. First, observational studies suggesting significant shifts in medical therapy should be succeeded by more stringent randomized clinical trials. When adjusting an independent outcome, such as cardiovascular disease, by multivariate regression, it’s imperative to include risk and protective factors. The conclusions might not apply broadly if a study’s participants are relatively uniform. Finally, researchers should carefully examine and detail in their manuscripts the statistical limitations inherent in their investigations.
While randomized trials are more rigorous than observational studies, even RCTs can be limited by adherence, attrition, short follow-up periods and lack of generalization to broader populations. This further emphasizes the need for caution when interpreting the results of nutrition studies since perfectly controlling diet over the long term is inherently challenging. The consensus among experts currently advocates for a balanced diet, exemplified by the Mediterranean Diet, which protects against cardiovascular issues and supports cancer prevention [42,43].
These evolving recommendations underscore the statistical advantages of employing concrete endpoints, such as diagnosed cardiovascular events, instead of surrogate endpoints, like cholesterol levels. Surrogate endpoints are measures that substitute for clinical endpoints of interest. For example, a study may use a change in blood pressure as a surrogate marker for the risk of stroke. Surrogate endpoints are convenient but can be misleading if the correlation with the clinical outcome is weak. Relying solely on them may overestimate clinical benefits or provide a false impression of efficacy. Hard clinical endpoints like mortality or cardiovascular events provide more definitive evidence. The everyday use of surrogate endpoints in dietary studies emphasizes caution when interpreting research that cannot comprehensively control for confounding variables.
Vaccinations and Autism
A study involving 12 children in 1998 suggested a potential link between autism and the Measles, Mumps and Rubella (MMR) vaccination [44]. This study was retracted in 2010, primarily due to ethical violations related to human subjects, but notably not for statistical errors that led to poorly supported and controversial findings [45]. In contrast, a comprehensive 2014 meta-analysis encompassing ten studies, which included data from over 1.2 million children, found no discernible association between the MMR vaccine and autism [46]. Nevertheless, concerns regarding a potential connection between the MMR vaccine and autism persist among some parents, contributing to a significant decline in MMR vaccination rates [47]. This underscores the critical importance of promptly identifying and addressing statistical errors to prevent the propagation of medical misinformation. Notably, the original 1998 study was hindered by a limited sample size, comprising only 12 children. Additionally, it lacked proper control groups and predominantly relied on parental recall. The fact that it took 12 years to retract this study and that it continues to influence vaccine hesitancy emphasizes the vital necessity of stringent statistical rigor before publication.
Arthroscopic Surgery for Knee Osteoarthritis
A randomized trial of 32 adults with moderate osteoarthritis found that arthroscopic knee surgery provided pain relief but was not superior to saline joint lavage alone [48]. A follow-up randomized, placebo-controlled study of 180 adults with osteoarthritis in 2002 found that neither arthroscopic nor lavage was superior to sham surgery [49]. These studies highlight the importance of considering the substantial placebo effects that can occur with invasive procedures [50].
Internal Mammary Artery Ligation for Angina Pectoris
Utilizing internal mammary artery ligation as a treatment for angina pectoris was widely accepted before the 1960s. This acceptance was based on a plausible hypothesis substantiated by an extensive study involving 304 patients [51,52]. An improvement was observed over a follow-up period ranging from 3 months to 4 years in 85% of the patients. However, it is essential to note that this study lacked a control group for comparative analysis, lacked blinding or randomization and did not conduct any statistical analysis of the results.
In contrast, a follow-up study conducted in 1960, though involving only 18 participants, offered a randomized, double-blind comparison of internal mammary artery ligation versus a sham operation [53]. In this study, all five participants who underwent sham surgery reported improvement, while nine out of thirteen who underwent internal mammary ligation demonstrated improvement. Nonetheless, it is noteworthy that this follow-up study also refrained from conducting a statistical analysis. Nevertheless, when subjected to the Fisher Exact test, the data yields a p-value of 0.28, in line with the authors’ conclusion that no discernible benefit was associated with internal mammary artery ligation.
Another study from the same era, albeit with a relatively small sample size of 17 participants, also employed a sham surgery approach and gained high credibility owing to its robust study design [54]. In this study, five participants in the ligation group experienced improvement, three worsened and one succumbed. In the sham group, five participants improved, two worsened and one succumbed. Once again, this study refrained from performing a statistical analysis. Nevertheless, when the results of these 17 participants were combined with those of the other sham surgery-controlled study, the Fisher Exact p-value equated to 0.48, further supporting the notion that internal mammary artery ligation did not confer any discernible benefit. Furthermore, it’s worth noting that the combined data has a robustness index of 5.75, consistent with robust statistical findings [55].
Additional subsequent studies have corroborated the results of the two-sham surgery-controlled studies, underscoring the importance of a rigorous study design. These studies also clearly demonstrated the potent placebo effect associated with invasive procedures.
Discussion
There are numerous pitfalls in the analysis of medical research studies. To advance medical science ethically, statistical rigor is required. This must be applied during study design, ethical review board analysis, data collection, data analysis and peer review. As the case studies show, errors in statistical analyses and their interpretation can have real-world adverse consequences. The ethical review board is the primary gatekeeper to ensure the ethical conduct of clinical trials, including its statistical analysis. In addition to ensuring the proper treatment of research subjects, these review boards are also tasked with evaluating the scientific merit of research studies. However, these review boards have shown substantial variation in their evaluations [56]. Thus, a primary consideration in improving future research is for ethical review boards to incorporate a more standardized and rigorous evaluation of statistical methodologies in their assessment of clinical trial proposals. Inevitably, despite careful oversight, statistical errors will occur in evaluating and interpreting research studies. In addition, honest disagreements over the proper statistical analysis of research findings occur. To help minimize these issues, journals can apply the open-access model to data. This allows post-publication peer review and can encourage thoughtful communication about the statistical analyses used by research studies [57]. Finally, to raise ethical standards in medical research, articles could specifically address the ethical implications of their findings. More and more journals require researchers to address study limitations in their discussion sections, which is a significant advancement because it requires a discussion of statistical shortcomings. However, ethical issues are typically limited to stating that an ethical review board approved the study. Ethical review board approval is the bare minimum standard of research ethics. It is better to directly consider the broader ethical implications beyond the numbers. For example, gene-editing technology research typically has profound ethical implications that go well beyond the statistical findings. These studies require careful analysis and discussion of the meaning and ethical implications of the statistical findings [58].
Conclusion
The proper application of statistical principles is fundamental for ethical medical research. The misapplication of statistical methods can directly violate moral tenets guiding medical research and hinder scientific progress. The reproducibility crisis in medical research can be resolved only by utilizing robust statistical techniques. Strategies like standardizing ethical review boards, making data open-access and requiring researchers to address ethical concerns promote the proper application of statistics. Through these and other efforts prioritizing statistical rigor, the research community can fulfill its ethical duty to produce meaningful research that consistently benefits patients and society.
Conflict of Interest
The author has no conflict of interest to declare.
References
- Ahmad D. Some applications of biostatistics to medical research. IJAR. 2019;7(2):28-31.
- An argument for divine providence, taken from the constant regularity observed in the births of both sexes. By Dr. John Arbuthnott, Physician in Ordinary to Her Majesty and Fellow of the College of Physicians and the Royal Society. Philosophical Transactions of the Royal Society of London. 1710;27(328):186-90.
- Lind J. A treatise of the scurvy, in three parts: containing an inquiry into the nature, causes and cure, of that disease. Cambridge University Press. 2014.
- Milne I. Who was James Lind and what exactly did he achieve. J R Soc Med. 2012;105(12):503-8.
- Gosztonyi K. How history of mathematics can help to face a crisis situation: the case of the polemic between Bernoulli and d’Alembert about the smallpox epidemic. Educational Studies in Mathematics. 2021;108(1-2):105-22.
- Laplace PS. A Philosophical Essay on Probabilites. New York: John Wiley and Sons. 1902.
- Kopf EW. Florence nightingale as statistician. Quarterly Publications of the American Statistical Association. 1916;15(116):388-404.
- Stanton JM. Galton, pearson and the peas: A brief history of linear regression for statistics instructors. J Statistics Edu. 2001;9(3).
- Otte A, Maier-Lenz H, Dierckx RA. Good clinical practice: historical background and key aspects. Nucl Med Commun. 2005;26(7):563-74.
- Huberty CJ. Historical origins of statistical testing practices. The J Experimental Edu. 1993;61(4):317-33.
- Grier DA. The origins of statistical computing. American Statistical Association. 2021 [Last accessed on: November 24, 2023].
https://ww2.amstat.org/asa175/statcomputing.cfm
- Norman GR, Streiner DL. PDQ Statistics (PDQ Series). 3rd Hamilton, ONT: PMPH USA. 2003.
- White S. Basic and clinical biostatistics. 5th New York: McGraw-Hill Education/Medical. 2019.
- Dahlberg SE, Korn EL, Le-Rademacher J, Mandrekar SJ. Clinical versus statistical significance in studies of thoracic malignancies. J Thorac Oncol. 2020;15(9):1406-8.
- Talbot D, Massamba VK. A descriptive review of variable selection methods in four epidemiologic journals: there is still room for improvement. Eur J Epidemiol. 2019;34(8):725-30.
- Heston TF. The percent fragility index. Int J Scientific Res. 2023;12(7):9-10.
- Schober P, Bossers SM, Schwarte LA. Statistical significance versus clinical importance of observed effect sizes: what do P values and confidence intervals really represent? Anesth Analg. 2018;126(3):1068-72.
- Goodman WM, Spruill SE, Komaroff E. A proposed hybrid effect size plus p -value criterion: empirical evidence supporting its use. The American Statistician. 2019;73(sup1):168-85.
- Stefan AM, Schönbrodt FD. Big little lies: a compendium and simulation of p-hacking strategies. R Soc Open Sci. 2023;10(2):220346.
- Heston TF. Standardizing predictive values in diagnostic imaging research. J Magn Reson Imaging. 2011;33(2):505.
- Sharma M. Errors in statistical modeling. (or why keep the human in the loop). Towards Data Science. 2021. [Last accessed on: November 24, 2023].
https://towardsdatascience.com/errors-in-statistical-modeling-c22978a98269
- Lee S, Lee DK. What is the proper way to apply the multiple comparison test? Korean J Anesthesiol. 2018;71(5):353-60.
- McGauran N, Wieseler B, Kreis J, Schüler YB, Kölsch H, Kaiser T. Reporting bias in medical research – a narrative review. Trials. 2010;11:37.
- Thomas R. Mitigating survivorship bias in scholarly research: 10 tips to enhance data integrity. Enago Academy. [Last accessed on: November 24, 2023].
https://www.enago.com/academy/survivorship-bias/
- The Decision Lab. Survivorship bias. The Decision Lab. 2020 [Last accessed on: November 26, 2023].
https://thedecisionlab.com/biases/survivorship-bias
- Andrade C. Confounding. Indian J Psychiatry. 2007;49(2):129-31.
- Zhou L, Zhao P, Wu D, Cheng C, Huang H. Time series model for forecasting the number of new admission inpatients. BMC Med Inform Decis Mak. 2018;18(1):39.
- Uyanto SS. Monte Carlo power comparison of seven most commonly used heteroscedasticity tests. Comm Stati Simul Comp. 2019;1-18.
- Joseph L, Bélisle P, Tamim H, Sampalis JS. Selection bias found in interpreting analyses with missing data for the prehospital index for trauma. J Clin Epidemiol. 2004 ;57(2):147-53.
- Dalal DK. Multi-collinearity in behavioral sciences research. In: Oxford research encyclopedia of business and management. Oxford University Press. 2023.
- Elliott HL. Post hoc analysis: use and dangers in perspective. J Hypertens Suppl. 1996;14(2):S21-4.
- Sbraga TP. Post hoc reasoning in possible cases of child sexual abuse: symptoms of inconclusive origins. Clin Psychol Sci Pract. 2003;10(3):320-34.
- Bonovas S, Piovani D. Simpson’s paradox in clinical research: A cautionary tale. J Clin Med. 2023;12(4).
- Peters DP, Ceci SJ. Peer-review practices of psychological journals: The fate of published articles, submitted again. Behav Brain Sci. 1982;5(02):187.
- Stampfer MJ, Colditz GA, Willett WC, Manson JE, Rosner B, Speizer FE, et al. Postmenopausal estrogen therapy and cardiovascular disease. Ten-year follow-up from the nurses’ health study. N Engl J Med. 1991;325(11):756-62.
- Rossouw JE, Anderson GL, Prentice RL, LaCroix AZ, Kooperberg C, Stefanick ML, et al. Risks and benefits of estrogen plus progestin in healthy postmenopausal women: principal results from the women’s health initiative randomized controlled trial. JAMA. 2002;288(3):321-33.
- Stöckli S, Koufatzidou M, Seehra J, Pandis N. The reporting of study limitations in randomized controlled trials published in the leading dental journals: Is it sufficient? J Dent. 2023;136:104603.
- Keys A, Anderson JT, Grande F. Prediction of serum-cholesterol responses of man to changes in fats in the diet. Lancet. 1957;273(7003):959-66.
- Central Committee for Medical and Community Program of the American Heart Association. Dietary fat and its relation to heart attacks and strokes. JAMA. 1961;175(5):389.
- Ramsden CE, Zamora D, Leelarthaepin B, Majchrzak-Hong SF, Faurot KR, Suchindran CM, et al. Use of dietary linoleic acid for secondary prevention of coronary heart disease and death: evaluation of recovered data from the Sydney Diet Heart Study and updated meta-analysis. BMJ. 2013;346:e8707.
- Hooper L, Martin N, Jimoh OF, Kirk C, Foster E, Abdelhamid AS. Reduction in saturated fat intake for cardiovascular disease. Cochrane Database Syst Rev. 2020;8(8):CD011737.
- Monllor-Tormos A, García-Vigara A, Morgan O, García-Pérez MÁ, Mendoza N, Tarín JJ, et al. Mediterranean diet for cancer prevention and survivorship. Maturitas. 2023;178:107841.
- Delgado-Lista J, Alcala-Diaz JF, Torres-Peña JD, Quintana-Navarro GM, Fuentes F, Garcia-Rios A, et al. Long-term secondary prevention of cardiovascular disease with a Mediterranean diet and a low-fat diet (CORDIOPREV): a randomised controlled trial. Lancet. 2022;399(10338):1876-85.
- Wakefield AJ, Murch SH, Anthony A, Linnell J, Casson DM, Malik M, et al. Ileal-lymphoid-nodular hyperplasia, non-specific colitis and pervasive developmental disorder in children. Lancet. 1998;351(9103):637-41.
- Retraction–Ileal-lymphoid-nodular hyperplasia, non-specific colitis and pervasive developmental disorder in children. Lancet. 2010;375(9713):445.
- Taylor LE, Swerdfeger AL, Eslick GD. Vaccines are not associated with autism: an evidence-based meta-analysis of case-control and cohort studies. Vaccine. 2014;32(29):3623-9.
- Thompson S, Meyer JC, Burnett RJ, Campbell SM. Mitigating vaccine hesitancy and building trust to prevent future measles outbreaks in England. Vaccines (Basel). 2023;11(2):288.
- Chang RW, Falconer J, Stulberg SD, Arnold WJ, Manheim LM, Dyer AR. A randomized, controlled trial of arthroscopic surgery versus closed-needle joint lavage for patients with osteoarthritis of the knee. Arthritis Rheum. 1993;36(3):289-96.
- Moseley JB, O’Malley K, Petersen NJ, Menke TJ, Brody BA, Kuykendall DH, et al. A controlled trial of arthroscopic surgery for osteoarthritis of the knee. N Engl J Med. 2002;347(2):81-8.
- Beecher HK. Surgery as Placebo. JAMA. 1961;176(13):1102.
- Battezzati M, Tagliaferro A, Cattaneo AD. Clinical evaluation of bilateral internal mammary artery ligation as treatment coronary heart disease. Am J Cardiol. 1959;4(2):180-3.
- Glover RP, Davila JC, Kyle RH, Beard JC, Trout RG, Kitchell JR. Ligation of the internal mammary arteries as a means of increasing blood supply to the myocardium. J Thoracic Surgery. 1957;34(5):661-78.
- Dimond EG, Kittle CF, Crockett JE. Comparison of internal mammary artery ligation and sham operation for angina pectoris. Am J Cardiol. 1960;5:483-6.
- Cobb LA, Thomas GI, Dillard DH, Merendino KA, Bruce RA. An evaluation of internal-mammary-artery ligation by a double-blind technic. N Engl J Med. 1959;260(22):1115-8.
- Heston TF. The robustness index: going beyond statistical significance by quantifying fragility. Cureus. 2023.
- Stark AR, Tyson JE, Hibberd PL. Variation among institutional review boards in evaluating the design of a multicenter randomized trial. J Perinatol. 2010;30(3):163-9.
- Grahe J. The necessity of data transparency to publish. J Soc Psychol. 2021;161(1):1-4.
- Ioannidis JPA. Why most published research findings are false. PLoS Med. 2005;2(8):e124.
This work is licensed under a Creative Commons Attribution 2.0 International License.
Author Info
Thomas F Heston1*
1Department of Medical Education and Clinical Sciences, Washington State University, Spokane, USA and Department of Family Medicine, University of Washington, Seattle, USA
*Correspondence author: Thomas F Heston, Department of Medical Education and Clinical Sciences, Washington State University, Spokane, USA and Department of Family Medicine, University of Washington, Seattle, USA; Email: [email protected]
Copyright
Copyright© 2023 by Heston TF. All rights reserved. This is an open access article distributed under the terms of the Creative Commons Attribution License, which permits unrestricted use, distribution, and reproduction in any medium, provided the original author and source are credited.
Citation
Citation: Heston TF. Statistical Ethics in Medical Research: A Narrative Review. Jour Clin Med Res. 2023;4(3):1-10.
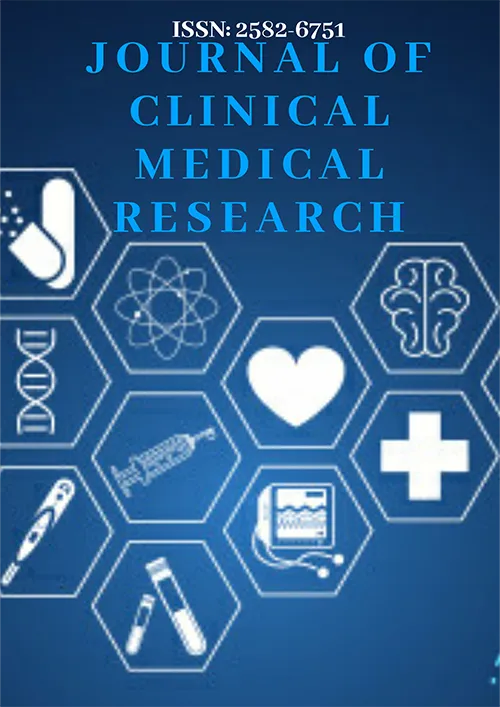